Big data, machine learning and artificial intelligence in clinical laboratory. Concepts and literature for education
Authors:
B. Friedecký
Authors‘ workplace:
Ústav klinické biochemie a diagnostiky, Fakultní nemocnice, Hradec Králové
Published in:
Klin. Biochem. Metab., 30, 2022, No. 3, p. 92-95
Overview
Working the big data needs using of artificial intelligence tools. This approach introduced currently into practice by large velocity leads to machine learning. Machine learning should be a strong way namely for the prediction of patient’s state, for precision medicine in oncology and many more cases. For example for aiming the real personalisation of patients in dese of their diagnosis and therapy. This work can be a helpful tool for the introduction of artificial intelligence in routine clinical laboratories.
Keywords:
artificial intelligence – machine learning – Big data
Sources
1. Xie, Y., Al-Aly, Z. Risks and burdens of incident diabetes in long COVID-19:a cohort study. Lancet Diabet. Endocrinol., 2022, 10(5), 311-321.
2. Al-Aly, Z., Xie, Y. High-dimensional characterization of post-acute sequelae of COVID-19. Nature, 2021, 594, 259–264.
3. Benke, K., Benke, G. Artificial Intelligence and Big Data in Public Health. Int. J Environ. Res. Public. Healt., 2018, 15(12), 2796.
4. Cabitza, F., Banfi, G. Machine learning in laboratory medicine-waiting for the flood? Clin. Chem. Lab. Med., 2017, 56(4), 516-524.
5. Cerriotti, F. Is there a classical role for the clinical laboratory digital health? Clin. Chem. Lab. Med., 2019, 57(3), 353-358.
6. Lippi, G., Plebani, M. Integrated diagnostics:the future of laboratory medicine? Biochem. Med. (Zagreb), 2020, 30(1), 18-30.
7. De Bruyne, S., Speeckaert, M. M., Van Biesen, W. M., Delanghe, J. R. Recent evolutions of machine learning applications in clinical laboratory medicine. Crit. Rev. Clin. Lab. Sci., 2020, 58(2),131-152.
8. Than, M. P., Pickering, J., Sandoval, Y. et al. Machine learning to Predict the Likelihood of Acute Myocardial Infarction. Circulation 2019, 140, 899-909
9. Gunčar, G., Kukan, M., Notar, M. et al. An application of machine learning to haematological diagnosis. Sci. Rep., 2018, 8, 411.
10. Cubukcu, H. C., Topcu, D. I., Bayraktar, N. et al. Detection of COVID-19by Machine Learning Using Routine Laboratory Tests. Am. J Clin. Pathol., 2022, 157(5), 758- 766.
11. Subramanian, M., Wojtusciszyn, A., Favre, L. a spol. Precision medicine in the era of artificial intelligence:implications in chronic disease management. J Transl. Med., 2020, 18, 472.
12. Bedoya, A. P., Futoma, J., Clement, M. E. et al. Machine learning for early detection of sepsis: an Internal and Temporal Validation study. JAMIA Open, 2020, 3(2), 252-260.
13. Komorowski, M., Celi, L. A., Badavi, O. et al. Artificial intelligence learns optimal treatment strategies for sepsis in intensive care. Nat. Med., 2018, 24(11), 1716-1720.
14. Vasey, B., Ursprung, S., Bedcloe, B. et al. Association of Clinician Diagnostic Performance with Machine Learning Based Decision Support Systems. A Systematic Rewiew. JAMA Network Open, 2021, 4, e211276
15. Ma, Ch., Wang, X., Wu, J. et al. Real-world-big data I laboratory medicine:Current status, application, and future considerations. Clin. Biochem., 2020, 84, 21-30.
16. Reita, J., Goto, T., Faridi, M. K. et al. Emergency Department triage prediction of clinical outcomes using machine learning models. Crit. Care, 2019, 23(1), 64.
17. Wang, H., Wang, H., Zhang, J. et al. Using machine learning to develop an autoverification system in clinical biochemistry laboratory, Clin. Chem. Lab. Med., 2020, 59(5), 883-891.
18. Froelich, M. F., Capoluongo, E., Kovacs, Z. et al. The value proposition of integrative diagnostics (early) detection of cancer. On behalf of the interdisciplinary Task and Finish Group “CNAPS/CTC”. Clin. Chem. Lab. Med., 2022, 60(6), 821-829.
19. Paranjape, K., Schinkel, M., Hammer, D. et al. The Value of Artificial Intelligence. Am. J Clin. Pathol. Lab. Med., 2021, 155(6), 823-831.
20. den Elzen, W. P. J., Brouwer, N., Thelen, M. H. et al. NUMBER:standardized reference intervals in the Netherlands using a big data approach. Clin. Chem. Lab. Med., 2018, 57(1), 42-56.
21. Ma, Ch., Xia, L., Chen, X. et al. Establishment of variation source and age-related reference interval model for 22 common biochemical analytes in older people assigned-world big data mining. Age Ageing, 2022, 49(6), 1062-1070.
22. Fleming, J. K., Katayev, A., Moorer, Ch. et al. Development of nation-wide reference intervals using an indirect method and harmonized assays. Clin. Biochem., 2022, 99, 20-59.
23. Yang, D., Su, Z., Zhao, M. Big data and reference intervals. Clin. Chim. Acta, 2022, 527, 23-32.
24. Ardou, O., Schmidt, R. L. Clinical laboratory employees attitudes toward artificial intelligence. Lab. Med., 2020, 51(6), 649-654.
25. Friedecký, B. Automatická strojová diagnostika-machine learning a precizní medicína. Pojmy, principy, perspektivy. Klin. Biochem. Metab., 2020, 4, 161-165.
Labels
Clinical biochemistry Nuclear medicine Nutritive therapistArticle was published in
Clinical Biochemistry and Metabolism
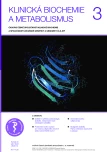
2022 Issue 3
Most read in this issue
- MxA protein and its application in early diagnosis of viral infections including SARS-CoV-2
- How we can put patients´ lives at risk together
- Galectin-3: an overview, pathophysiology, analytics, limits of interpretation and biological variation.
- Biological variability. Concepts and literature recommended to education