Predicting patient survival after deceased donor kidney transplantation using flexible parametric modelling
Background:
The influence of donor and recipient factors on outcomes following kidney transplantation is commonly analysed using Cox regression models, but this approach is not useful for predicting long-term survival beyond observed data. We demonstrate the application of a flexible parametric approach to fit a model that can be extrapolated for the purpose of predicting mean patient survival. The primary motivation for this analysis is to develop a predictive model to estimate post-transplant survival based on individual patient characteristics to inform the design of alternative approaches to allocating deceased donor kidneys to those on the transplant waiting list in the United Kingdom.
Methods:
We analysed data from over 12,000 recipients of deceased donor kidney or combined kidney and pancreas transplants between 2003 and 2012. We fitted a flexible parametric model incorporating restricted cubic splines to characterise the baseline hazard function and explored a range of covariates including recipient, donor and transplant-related factors.
Results:
Multivariable analysis showed the risk of death increased with recipient and donor age, diabetic nephropathy as the recipient’s primary renal diagnosis and donor hypertension. The risk of death was lower in female recipients, patients with polycystic kidney disease and recipients of pre-emptive transplants. The final model was used to extrapolate survival curves in order to calculate mean survival times for patients with specific characteristics.
Conclusion:
The use of flexible parametric modelling techniques allowed us to address some of the limitations of both the Cox regression approach and of standard parametric models when the goal is to predict long-term survival.
Keywords:
Kidney transplantation, Survival, Multivariable analysis, Flexible parametric model, Extrapolation
Autoři:
Bernadette Li 1*; John A. Cairns 1; Matthew L. Robb 2; Rachel J. Johnson 2; Christopher J. E. Watson 3; John L. Forsythe 4; Gabriel C. Oniscu 4; Rommel Ravanan 5; Christopher Dudley 5; Paul Roderick 6; Wendy Metcalfe 7; Charles R. Tomson 8; J. Andrew Bradley 3
Působiště autorů:
Department of Health Services Research and Policy, London School of Hygiene and Tropical Medicine, 15-1 Tavistock Place, London WC1H 9SH, UK.
1; NHS Blood and Transplant, Bristol, UK.
2; Department of Surgery, University of Cambridge and the NIHR Cambridge Biomedical Research Centre, Cambridge, UK.
3; Transplant Unit, Royal Infirmary of Edinburgh, Edinburgh, UK. 5Richard Bright Renal Unit, Southmead Hospital, Bristol, UK.
4; Primary Care and Population Sciences, Faculty of Medicine, University of Southampton, Southampton, UK.
6; Scottish Renal Registry, Glasgow, UK.
7; Department of Renal Medicine, Freeman Hospital, Newcastle upon Tyne, UK.
8
Vyšlo v časopise:
BMC Nefrol 2016, 17:51
Kategorie:
Technical advance
prolekare.web.journal.doi_sk:
https://doi.org/10.1186/s12882-016-0264-0
© Li et al. 2016
Open Access This article is distributed under the terms of the Creative Commons Attribution 4.0
International License (http://creativecommons.org/licenses/by/4.0/), which permits unrestricted use, distribution, and reproduction in any medium, provided you give appropriate credit to the original author(s) and the source, provide a link to the Creative Commons license, and indicate if changes were made. The Creative Commons Public Domain Dedication waiver (http://creativecommons.org/publicdomain/zero/1.0/) applies to the data made available in this article, unless otherwise stated.
The electronic version of this article is the complete one and can be found online at: http://bmcnephrol.biomedcentral.com/articles/10.1186/s12882-016-0264-0
Souhrn
Background:
The influence of donor and recipient factors on outcomes following kidney transplantation is commonly analysed using Cox regression models, but this approach is not useful for predicting long-term survival beyond observed data. We demonstrate the application of a flexible parametric approach to fit a model that can be extrapolated for the purpose of predicting mean patient survival. The primary motivation for this analysis is to develop a predictive model to estimate post-transplant survival based on individual patient characteristics to inform the design of alternative approaches to allocating deceased donor kidneys to those on the transplant waiting list in the United Kingdom.
Methods:
We analysed data from over 12,000 recipients of deceased donor kidney or combined kidney and pancreas transplants between 2003 and 2012. We fitted a flexible parametric model incorporating restricted cubic splines to characterise the baseline hazard function and explored a range of covariates including recipient, donor and transplant-related factors.
Results:
Multivariable analysis showed the risk of death increased with recipient and donor age, diabetic nephropathy as the recipient’s primary renal diagnosis and donor hypertension. The risk of death was lower in female recipients, patients with polycystic kidney disease and recipients of pre-emptive transplants. The final model was used to extrapolate survival curves in order to calculate mean survival times for patients with specific characteristics.
Conclusion:
The use of flexible parametric modelling techniques allowed us to address some of the limitations of both the Cox regression approach and of standard parametric models when the goal is to predict long-term survival.
Keywords:
Kidney transplantation, Survival, Multivariable analysis, Flexible parametric model, Extrapolation
Zdroje
1. Morris PJ, Johnson RJ, Fuggle SV, Belger MA, Briggs JD. Analysis of factors that affect outcome of primary cadaveric renal transplantation in the UK. HLA Task Force of the Kidney Advisory Group of the United Kingdom Transplant Support Service Authority (UKTSSA). Lancet. 1999;354:1147–52.
2. Fuggle SV, Allen JE, Johnson RJ, Collett D, Mason PD, Dudley C, et al. Factors affecting graft and patient survival after live donor kidney transplantation in the UK. Transplantation. 2010;89:694–701.
3. Johnson RJ, Fuggle SV, O'Neill J, Start S, Bradley JA, Forsythe JL, et al. Factors influencing outcome after deceased heart beating donor kidney transplantation in the United Kingdom: an evidence base for a new national kidney allocation policy. Transplantation. 2010;89:379–86.
4. Summers DM, Johnson RJ, Allen J, Fuggle SV, Collett D, Watson CJ, et al. Analysis of factors that affect outcome after transplantation of kidneys donated after cardiac death in the UK: a cohort study. Lancet. 2010;376:1303–11.
5. Summers DM, Johnson RJ, Hudson A, Collett D, Watson CJ, Bradley JA. Effect of donor age and cold storage time on outcome in recipients of kidneys donated after circulatory death in the UK: a cohort study. Lancet. 2013;381:727–34.
6. Royston P, Lambert PC. Flexible Parametric Survival Analysis Using Stata: Beyond the Cox Model. College Station, Texas: Stata Press; 2011.
7. Baskin-Bey ES, Kremers W, Nyberg SL. A recipient risk score for deceased donor renal allocation. Am J Kidney Dis. 2007;49:284–93.
8. Wolfe RA, McCullough KP, Schaubel DE, Kalbfleisch JD, Murray S, Stegall MD, et al. Calculating life years from transplant (LYFT): methods for kidney and kidney-pancreas candidates. Am J Transplant. 2008;8:997–1011.
9. Concepts for kidney allocation 2011, Organ Procurement and Transplantation Network.
10. A Guide to Calculating and Interpreting the Estimated Post-Transplant Survival (EPTS) Score Used in the Kidney Allocation System (KAS). https://optn.transplant.hrsa.gov/media/1511/guide_to_calculating_interpreting_epts.pdf. Accessed 8 May 2014.
11. Israni AK, Salkowski N, Gustafson S, Snyder JJ, Friedewald JJ, Formica RN, et al. New national allocation policy for deceased donor kidneys in the United States and possible effect on patient outcomes. J Am Soc Nephrol. 2014;25:1842–8.
12. Latimer N, NICE DSU. Technical Support Document 14: Undertaking survival analysis for economic evaluations alongside clinical trials - extrapolation with patient-level data. 2011. http://www.nicedsu.org.uk. Accessed 8 Dec 2014.
13. Royston P, Parmar MK. Flexible parametric proportional-hazards and proportional-odds models for censored survival data, with application to prognostic modelling and estimation of treatment effects. Stat Med. 2002;21:2175–97.
14. Pruthi R, Hilton R, Pankhurst L, Mamode N, Hudson A, Roderick P, et al. UK Renal Registry 16th annual report: chapter 4 demography of patients waitlisted for renal transplantation in the UK: national and centre-specific analyses. Nephron Clin Pract. 2013;125:81–98.
15. Johnson RJ, Fuggle SV, Mumford L, Bradley JA, Forsythe JL, Rudge CJ. A New UK 2006 National Kidney Allocation Scheme for deceased heartbeating donor kidneys. Transplantation. 2010;89:387–94.
16. Collett D. Modelling Survival Data in Medical Research 2nd edition ed. London: Chapman & Hall/CRC; 2003.
17. Lambert PC, Royston P. Further development of flexible parametric models for survival analysis. Stata J. 2009;9:265–90.
18. Harrell Jr FE, Lee KL, Mark DB. Multivariable prognostic models: issues in developing models, evaluating assumptions and adequacy, and measuring and reducing errors. Stat Med. 1996;15:361–87.
19. Wolfe RA, McCullough KP, Leichtman AB. Predictability of survival models for waiting list and transplant patients: calculating LYFT. Am J Transplant. 2009;9:1523–7.
20. Watson CJ, Johnson RJ, Birch R, Collett D, Bradley JA. A simplified donor risk index for predicting outcome after deceased donor kidney transplantation. Transplantation. 2012;93:314–8.
Štítky
Detská nefrológia NefrológiaČlánok vyšiel v časopise
BMC Nephrology
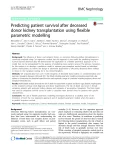
2016 Číslo 51
- I „pouhé“ doporučení znamená velkou pomoc. Nasměrujte své pacienty pod křídla Dobrých andělů
- Aktuálne európske odporúčania pre liečbu renálnej koliky v dôsledku urolitiázy
- MUDr. Šimon Kozák: V algeziológii nič nefunguje zázračne cez noc! Je dôležité nechať si poradiť od špecialistov
Najčítanejšie v tomto čísle