DESIGN OF AN EXPERIMENTAL LASER SPECKLE CONTRAST IMAGING SYSTEM AND IMAGE
Laser speckle contrast imaging (LSCI) is a noninvasive method, which uses scattered light to estimate the flow of a fluid containing scatterers. Thus, it can be especially used to estimate blood flow. Laser light is randomly scattered on the tissue and this refracted light interfere with reflected light, giving birth to laser speckle noise. As the light scatters on moving red blood cells, the speckle pattern is blurred due to the exposition time. This blurring is a function of velocity which can be estimated from the degree of blur, termed as speckle contrast. Therefore, blood cells act like contrast agent, outlining blood vessels. The simple setup, unnecessary contrast agent and unharming nature are making LSCI a popular tool for studying blood flow dynamics and vascular structure. This paper presents a new, simple experimental setup and image processing methods to treat laser speckle images aiming to estimate relative blood flow and vascular structure.
Keywords:
LSCI, laser speckle contrast imaging, blood flow, speckle noise
Authors:
Branislav Hesko; Vratislav Harabiš; Radim Kolář
Authors‘ workplace:
Department of Biomedical Engineering, BUT, Brno, Czech Republic
Published in:
Lékař a technika - Clinician and Technology No. 3, 2017, 47, 101-107
Category:
Original research
Overview
Laser speckle contrast imaging (LSCI) is a noninvasive method, which uses scattered light to estimate the flow of a fluid containing scatterers. Thus, it can be especially used to estimate blood flow. Laser light is randomly scattered on the tissue and this refracted light interfere with reflected light, giving birth to laser speckle noise. As the light scatters on moving red blood cells, the speckle pattern is blurred due to the exposition time. This blurring is a function of velocity which can be estimated from the degree of blur, termed as speckle contrast. Therefore, blood cells act like contrast agent, outlining blood vessels. The simple setup, unnecessary contrast agent and unharming nature are making LSCI a popular tool for studying blood flow dynamics and vascular structure. This paper presents a new, simple experimental setup and image processing methods to treat laser speckle images aiming to estimate relative blood flow and vascular structure.
Keywords:
LSCI, laser speckle contrast imaging, blood flow, speckle noise
Introduction
Blood flow mapping is an important part of the diagnostics procedure. Usually, Doppler-based methods are widely used [1]. These methods are using reflected wave frequency shift to estimate blood flow [2]. Recently, a new technique has been widely explored, but its roots date back to the last century and is termed as laser speckle contrast imaging [1–4]. It is full field method based on light scattering on small objects. The main advantages of this method are low-cost instrumentation and high spatial and temporal resolution compared to original Doppler methods [4]. Moreover, it has been shown, that the method is also reproducible [5].
In Doppler-based methods, the temporal resolution is limited by wave propagation velocity, while the limitation of the LSCI lays in camera properties [6]. Using fast ICCD (intensified charge-coupled device) cameras allows temporal resolution higher than blood flow changes due to the heart rate [7]. In addition, spatial resolution in Doppler systems is given by wavelength, while the spatial resolution of the LSCI system is determined by the optical system. The main drawback of the laser system lies in the laser light absorption by skin or other tissue, resulting in shallow penetration rate [8]. Thus, the main field of use of the LSCI system could be skin repletion screening or retina blood flow monitoring [8, 9].
The laser light enlightens tissue and is scattered on the object, which size is comparable to the wavelength of light. Scattered beams interfere with reflected or incident beams, resulting in forming speckle-noise patterns [10]. When the scatterers are mobile, the speckle pattern becomes blurred. This is caused by time integration of the speckle patterns as the exposure time is always finite [7]. It is possible to relate blood flow to a position in the image by transforming original images into contrast images, using equation [9]:
where µN is local mean value and σN is local standard deviation of the pixel intensities in a predefined neighborhood. K(x,y) is the contrast value at position (x,y).
The size N of the neighborhood used to compute mean and standard deviation is a compromise between spatial precision and blood flow continuity. If the region neighborhood is taken too small, the speckle pattern has the form of small regions and supplementary post-processing is needed to make blood flow continuous in the vessel. On the other hand, if the neighborhood is too large, spatial information is lost, the vessel contour will become unclear [6, 11]. The relation between contrast image based on computing speckle blurring is not linear [3]. Therefore, more often, decorrelation time value is used [3, 9]. The relation between contrast value and decorrelation time is the following [11]:
where T is exposure time in milliseconds, τ is decorrelation time also in milliseconds and K represents contrast value. This equation is not linear and not easy to analytically solve. The contrast value is a function of the ratio of decorrelation time and exposition time [11]. Thus, two measurements are comparable only if their exposition time is constant [3, 12].
As we will see later, system specific variables are also present, which prevents from different system measurement comparison [3, 9]. It has been proven [13], that also air movement during acquisition could lead to different results. Moreover, it is possible to deduce that contrast values have no units, as the only variable is the ratio, which is dimensionless.
Measurement
For the measurement, we have designed a new, simple experimental system, see Figure 1, which mainly relies on a capillary of the size of 5 mm wide and 0.5 mm deep where the fluid flows and creates blurred speckle pattern.
As a fluid, we are using water with microparticles. The most important properties of the microparticles are their size and material, which should reflect or absorb incident light at the chosen wavelength. We are using scatterers made of latex and their size is 1.1 μm, prepared by SIGMA manufacturer with product name LB11-15ML. The size is important because speckle pattern size is proportional to the microparticles size. Microparticles with smaller size could be unnoticeable and moreover, at the nanometer level, the diffraction limit of light does not allow to distinguish particles. On the contrary, big particles could cause loss of positional precision. The flow of the fluid is secured using peristaltic pump Dynamax RP-1, which prevents from absolute measurement, as we are not able to estimate correct value of the flow. The only parameter of the pump is the number of turns per minute, which does not allow absolute flow measurement. For that reason, in later stages, we used injection pump NE-1010, which allows direct flow setup, but only for a limited amount of time. We have used the following flows (0.075, 0.75 and 7.5) ml/min and exposition times (5, 10, 15, 20) ms. The capillary is enlightened by a tunable laser. We are using commercially available laser diode made by ThorLabs, model 658P040. This diode is controlled by ThorLabs LDC 205C laser diode controller, which sets up current through the diode, thus laser light power. We must secure uniform enlightenment because as we will show later, the light distribution influences the value of the contrast. The most important parameter of our laser is the power, which can be tuned. In this experimental system, we usually use 30 mW of laser power. This value is three orders of magnitude larger, then the maximal acceptable incident light power onto the retina. But, for other applications, like vessel mapping under the skin, the laser power is under limits. The last part of our system is video camera from ImagingSource, model number DMK-21F04 with professional lens Navitar. For the measurement, the following video settings are used. The frame size is 720×480, 60 frames per second and typically 30 seconds of recording time. Camera controlling software declares capability to record sequences at 100 frames per second, but the set value is sufficient. All the images were processed offline. To make the measurement real time, some processing modifications are needed.
Image processing
Image processing is divided into two distinct tasks. Relative flow visualization and vessel delineation.
For different fluid velocities, we have acquired videos of the length of approximately 30 s. Then, we have designed the video processing software. An example of an acquired raw image is shown on the image in Fig. 2.
Firstly, it is possible to see, that there is no significant difference in pixel intensities and thus, thresholding is not possible. The difference between vessel content and its surroundings lays in speckle statistics. After processing the images, using equation (1), the result is a contrast image, where the vessel content is clearly different from the background. As mentioned in the introduction, the choice of the neighborhood of the vessel is an important parameter for diagnostics.
As it is necessary to maintain spatial information, the neighborhood is chosen typically of size 9–15 pixels depending on the desired smoothness. To make images smoother, time averaging is used. It is important to notice, that the length of the averaging time filter must be smaller than significant changes in the flow in time. Otherwise, information may be lost. Especially, when using this technique in vascular diagnostics, the heartbeat frequency is important, which varies in a range 1–3 Hz. For these reasons, filter length is chosen mostly in interval 9–15 pixels. Moreover, to reduce induced spatial errors from the time filter, a spatial averaging filter of size 5 is applied. Thanks to the speckle pattern properties, the flow of fluid is clearly visible. This is evident in Fig. 3.
The decorrelation time has been introduced as more precise and quantitative measure of blood flow, as it is more respecting nonlinear dependence of the blood flow on speckle pattern. Transforming the equation (2) into [4], one obtains:
β is a system dependent constant which is usually set to one. K represents contrast value and is a function of exposure time and decorrelation time τ. x is an unknown variable which is equal to x = T / τ. Solving this equation for known values of K will get a transformation relation to obtain τ.
This equation will serve to transform contrast values into decorrelation time images, which will be a more linear measure of blood flow. First, general maximal and minimal contrast values from all images of the video are extracted. In this range given by maximal and minimal value, the equation (3) is numerically solved. It should be solved separately for every pixel intensity value or, to speed up the process, minimal and maximal value are used and a lookup table in this interval for 1000 values is computed. Each pixel intensity value is then transformed using this lookup table. To solve the equation, fsolve function from the Python’s SciPy module is used. This function implements modified Powell hybrid method. The solution of the equation is stable in the range [0, 1). For values close to one, the solution diverges. For values near zero, the solution converges to zero value of the decorrelation time. The dependence of inverse decorrelation time as a function of the contrast value for different exposition times is shown in Fig. 4.
Vessel detection
An important part of the processing is vessel detection and delimiting its borders. This step is needed because we want to show velocities only in vessels, not background. In real tissue we are not concerned about decorrelation time values outside the vessels. First, mean speckle image, from all the contrast images in a video is created, which will be used to delineate vessel borders, thus averaging occurs in time. The image, in pseudocoloring, is shown as Fig. 5. It is possible to distinguish the vessel from its surroundings because speckle contrast is high in the middle of the vessel due to the scatterers movement. As these scatterers are moving in a common direction and we have finite exposition time, the speckles have become blurred. Using statistics, we have detected this blurring, resulting in relative velocity mapping.
Next, inverse binary thresholding with a threshold determined using Otsu method is applied to the mean time contrast speckle vessel imaging. This step enhances the vessel and assigns zero value to the background. All the pixels belonging to the vessel are assigned the value of one. Due to the presence of noise, the vessel contour is blurred and imprecise. Further processing is needed to achieve satisfactory vessel border delineation, so we implemented following steps:
- Dilatation of the thresholded image with a one-dimensional mask of size 15 in horizontal and vertical direction. The result is saved as two distinct images.
- Morphological closing in both images to fill small pixel holes, to make the detected vessel compact.
- Labeling connected components.
- Assuming, that the biggest object represents the vessel, picking up the biggest object from the horizontally and vertically dilated image.
- Vessel axis detection and smoothing it, using B-splines, because we suppose, that middle position should be a continuous function in space.
- Primary border is detected as the first point with value of one in concerned direction.
- Finally, the last step consists of a vessel border detection. The border location is computed as a weighted average of the middle point and adjacent points of the primary border. Also, the assumption, that the lower and upper border of the vessel is equally distant from the middle is applied.
Finally, the resulting border is written in all images of the video. Example of a time average speckle contrast image with written border is inserted in Fig. 6.
It is necessary to notice, that choosing only the biggest object could eventually lead to losing information if the vessel narrows in some place. In that case, the object, representing the vessel will become discontinuous leading to splitting into two distinct objects. Only one of them would be then detected. To treat real vessel images, this processing should be adjusted to treat more than just one object from the image.
Results and discussion
Videos have been acquired for different speeds of the fluid. We have calculated decorrelation time images and together with the vessel position, they have been put together, to form result videos.
Inside our model vessel, the speed of the fluid is pseudocolored by hot pseudocoloring, which means, that the velocity of the content is greater than the velocity of the background because it is more red. Moreover, our model shows linear fluid movement, in other words, the velocity is not dependent on the radial position in the vessel. This is proven in Fig. 7.
Afterwards, instead of the peristaltic pump, we used an infusion pump, where we know directly the value of the velocity. For different fluid flows and exposure times, we have computed mean contrast value in a delineated capillary, which is a function of velocity. In the same Fig. 8, we have inserted also mean image value, which, should be a function of velocity too, because the background is constant of inverse contrast.
Based on Fig. 8, we can conclude, that the inverse contrast value, which is a function of particle velocity increases with fluid flow. For exposition time of 5 ms, the difference in inverse contrast value between flows of 0.75 ml/min and 7.5 ml/min is not evident, as when comparing them with the smallest fluid flow. For higher exposition time of 15 ms, the velocity increases as it was expected. More experiments will be needed to estimate the exact shape of inverse decorrelation time, respectively inverse contrast value as a function of microparticles velocity.
Microparticles, after some time, tend to form clusters. These clusters remain blocked in the capillary and are not moving. Thus, in the resulting videos, this object should not have been labelled as moving. This fact is visible mostly in Figure 4.
Laser illumination is one of the key features, and non-uniform illumination will cause a higher value of the contrast in image, resulting in an impression of moving background. Later, we will have to include an algorithm for adaptive brightness correction.
Original images are loaded with speckle noise. This noise lowers spatial resolution and causes problems when detecting vessel axis and borders. The presented algorithm is partially noise robust and small artefact fragments, like on figure 6, for example from the clusters of microparticles are ignored in detection. This is secured by use of morphological operations, dilatation and closing. Robustness is also caused by using B-splines to fit values of the vessel axis. After detecting the middle as the half distance between the top and bottom point of the thresholded image, the middle is given by a set of points, which are independent to their neighbourhood. An image of the initially detected middle is attached below as Fig. 8.
From the Figure 9, we can deduce, that the border detected as the first white pixel and the last white pixel in the appropriate row/column would be noisy and would not respect continuity of the vessel border. This problem is solved by using adjacent points of the border pixel as well as the corresponding B-spline fitted middle point and corresponding pixels from the opposite border. The equal distance from the middle to both borders is respected too.
Lastly, it is possible to compare only videos with the same measurement settings. Only one parameter could be varied. In most cases, we are changing velocity and comparing resulting decorrelation time intensity. We suppose, that with increasing velocity, the intensity in the vessel will raise. To achieve this step, contrast images normalization by dividing by the maximum value must be avoided and only dividing by the maximum value from the video with highest fluid velocity should be settled. The final resulting image with delimited border and middle in form of relative speed map is inserted as Fig. 10.
As we can see from the final image, the method is quite robust and can handle noise relatively well. The flow is, according to the visual scene, constant and not dependent on radial position. Latex clusters are correctly assigned as non-moving, but they bring problems to the vessel detection. The speed difference between the vessel and background is clearly significant, so we can conclude the justification of our method.
Conclusion
In this paper we have presented experimental system for LSCI method investigation and sufficient methods for data processing. The LSCI system is composed of a simple measurement system, followed by image processing and video treating. The relation between inverse contrast valued images given by equation (1) and fluid flow velocity has been shown in Fig. 8, just like the dependency of the decorrelation time on the fluid flow.
For a better understanding of the relationship between inverse decorrelation time (inverse contrast), more measurements are needed. We found out various problems, which are common to all LSCI systems. We think, that it will be easier to resolve them on our experimental system, because of its simplicity and easy settings influence studying. There are still some persistent problems caused by latex particles having tendencies to clustering. For real-time measurements, which is needed to invivo examination, the algorithm has to speeded up by using different programming language and parallel processing because some parts of the algorithm are independent, thus distributable.
In the following work, we will modify our experimental system to a measurement of two different velocities in opposite directions. This system will be able to better simulate LSCI method in a living tissue and hopefully clarify hidden relationships between blurring and particles velocity. In the future, we will investigate the possibility to use this method together with a fundus camera. This step will bring various problems, mostly connected with the low laser light intensity because maximal unharming power is at about P = 0.1 mW. Moreover, LSCI assumes static scene, which is not always the case in fundus camera screening, as it is often necessary to fuse images.
This system brings a unique possibility to achieve absolute velocity measurements, which is not possible in the state of art systems. We will be obliged to measure calibration curve, while maintaining constant conditions. The influence of the light on the resulting velocity and the exact relation between velocity and blurring is to be determined and examined.
Finally, we will try to investigate the blood flow measurement in the vessels lying at the top levels of the skin. Deeper investigations are not possible, because of the low penetration of the laser light through the skin. This could lead to a simple, cheap and efficient tool for skin vascularisation diagnostics.
Acknowledgement
The work was not supported by a research grant or contract.
Branislav Hesko
Department of Biomedical Engineering
Faculty of Electrical Engineering and Communication
Brno University of Technology
Technická 12, CZ-616 00 Brno
E-mail: xhesko00@stud.feec.vutbr.cz
Phone: +420 778 788 954
Vratislav Harabiš
Department of Biomedical Engineering
Faculty of Electrical Engineering and Communication
Brno University of Technology
Technická 12, CZ-616 00 Brno
E-mail: harabis@feec.vutbr.cz
Radim Kolář
Department of Biomedical Engineering
Faculty of Electrical Engineering and Communication
Brno University of Technology
Technická 12, CZ-616 00 Brno
Sources
[1] Madsen, S.: Optical Methods and Instrumentation in Brain Imaging and Therapy: 3 (Bioanalysis). Springer, 2012, 280 p, ISBN: 978-1-4614-4978-2.
[2] Fercher, A. F. and Briers, J. D.: Flow visualization by means of single-exposure speckle photography. Optics Communications 37(5), 1981, p. 326–330.
[3] Briers, D., Duncan, D. D. et al.: Laser speckle contrast imaging: theoretical and practical limitations. Journal of Biomedical Optics 18(6), june 2013, 10 p.
[4] Millet, C. et al.: Comparison between laser speckle contrast imaging and laser doppler imaging to assess skin blood flow in humans. Microvascular Research 82/2, 2011, p. 147–151.
[5] Roustit, M. et al.: Excelent reproducibility of laser speckle contrast imaging to assess skin microvascular reactivity. Mikrovascular Research 80(3), 2010, p. 505–511.
[6] Kirkpatrick, S.J et al.: Detrimental effects of speckle-pixel size matching in laser speckle contrast imaging. Optics letters, 33(24):2886, dec. 2008.
[7] Dunn, A. K.: Speckle contrast imaging of cerebral blood flow. Annals of Biomedical Engineering 40(2), p. 367–377, nov. 2011.
[8] Cheng, H., Yan, Y. and Duong, T. Q.: Temporal statistical analysis of laser speckle images and its application to retinal blood-flow imaging. Optics Express 16(14), 2008.
[9] Boas, D.A and Dunn, A.K. Laser speckle contrast imaging in biomedical optics, Journal of Biomedical Optics, 2010.
[10] Cheng, H and Duong, T. Q.: Simplified laser-speckle-imaging analysis method and its application to retinal blood flow imaging. Optics Letters 32(15), 2007.
[11] Richards, L. M. et al.: Low-cost laser speckle contrast imaging of blood flow using a webcam. Biomedical Optics Express 4(10), 2013, p. 2269–2283.
[12] Yuan, S., Devor, A., Boas, A. and Dunn, A. K.: Determination of optimal exposure time for imaging blood flow changes with laser speckle contrast imaging. Applied Optics, 44(10), 2005, p. 18–23.
[13] Mahé, G. et al.: Air movements interfere with laser speckle contrast imaging recordings. Laser in medical science, 27(5), 2012, p. 1073–1076.
Labels
BiomedicineArticle was published in
The Clinician and Technology Journal
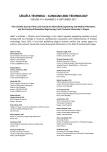
2017 Issue 3
Most read in this issue
- DEVELOPMENT OF NEW TECHNIQUE FOR ACCURATE WEAR ANALYSIS OF EXPLANTED TOTAL HIP REPLACEMENTS
- RELIABILITY AND SOURCE OF ERRORS IN END-TIDAL GAS CONCENTRATION EVALUATION ALGORITHMS DURING AVALANCHE SNOW AND REBREATHING EXPERIMENTS
- DESIGN OF AN EXPERIMENTAL LASER SPECKLE CONTRAST IMAGING SYSTEM AND IMAGE
- DETERMINATION OF THE GEOMETRICAL AND VISCOELASTIC PROPERTIES OF SCAFFOLDS MADE BY ADdITIVE MANUFACTURING USING BIOPLOTTER